【佳學(xué)基因檢測(cè)】癌癥建模:動(dòng)態(tài)系統(tǒng)中“組學(xué)”信息的整合
上海腫瘤基因檢測(cè)機(jī)構(gòu)機(jī)會(huì)
參加學(xué)術(shù)會(huì)議時(shí)了解《J Bioinform Comput Biol》在?2007 Aug;5(4):977-86發(fā)表了一篇題目為《癌癥建模:動(dòng)態(tài)系統(tǒng)中“組學(xué)”信息的整合》腫瘤靶向藥物治療基因檢測(cè)臨床研究文章。該研究由Beatriz Stransky,?Junior Barrera,?Lucila Ohno-Machado,?Sandro J De Souza等完成。促進(jìn)了腫瘤的正確治療與個(gè)性化用藥的發(fā)展,進(jìn)一步強(qiáng)調(diào)了基因信息檢測(cè)與分析的重要性。
腫瘤靶向藥物及正確治療臨床研究?jī)?nèi)容關(guān)鍵詞:
0
腫瘤靶向治療基因檢測(cè)臨床應(yīng)用結(jié)果
過去 10 年見證了許多技術(shù)的興起,這些技術(shù)從許多生物中產(chǎn)生了前所未有的基因組規(guī)模數(shù)據(jù)。盡管研究界已經(jīng)成功地探索了這些數(shù)據(jù),但仍然存在許多挑戰(zhàn)。其中之一是將這些數(shù)據(jù)集有效地直接集成到基于生物系統(tǒng)數(shù)學(xué)建模的方法中。在癌癥中的應(yīng)用就是一個(gè)很好的例子。癌癥信息和建模之間的橋梁可以通過兩種主要類型的互補(bǔ)策略來實(shí)現(xiàn)。首先,有一種自下而上的方法,其中數(shù)據(jù)生成有關(guān)給定系統(tǒng)組件之間的結(jié)構(gòu)和關(guān)系的信息。此外,還有一種自上而下的方法,其中控制論和系統(tǒng)理論知識(shí)用于創(chuàng)建描述系統(tǒng)機(jī)制和動(dòng)態(tài)的模型。這些方法也可以與產(chǎn)生結(jié)合詳細(xì)機(jī)制和廣泛生物學(xué)范圍的多尺度模型聯(lián)系起來。在這里,我們給出了該領(lǐng)域的總體情況,并討論了應(yīng)對(duì)未來主要挑戰(zhàn)的可能策略。
腫瘤發(fā)生與反復(fù)轉(zhuǎn)移國際數(shù)據(jù)庫描述:
The last 10 years have seen the rise of many technologies that produce an unprecedented amount of genome-scale data from many organisms. Although the research community has been successful in exploring these data, many challenges still persist. One of them is the effective integration of such data sets directly into approaches based on mathematical modeling of biological systems. Applications in cancer are a good example. The bridge between information and modeling in cancer can be achieved by two major types of complementary strategies. First, there is a bottom-up approach, in which data generates information about structure and relationship between components of a given system. In addition, there is a top-down approach, where cybernetic and systems-theoretical knowledge are used to create models that describe mechanisms and dynamics of the system. These approaches can also be linked to yield multi-scale models combining detailed mechanism and wide biological scope. Here we give an overall picture of this field and discuss possible strategies to approach the major challenges ahead.
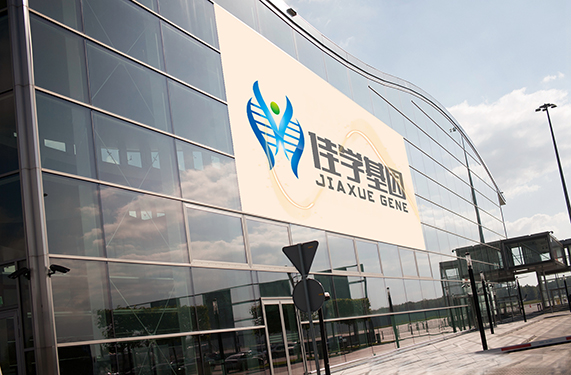
(責(zé)任編輯:佳學(xué)基因)